【】大胆但是预测因為模型的原因
大膽預測一下2022卡塔爾世界杯小組賽(世界杯2022世界杯時間)_世界杯 ( 性為,大胆算法 )
www.ty42.com 日期:2022-12-12 00:00:00| 評論(已有356407條評論) 波盈體育小編整理了以下關於 [ 大膽預測一下2022卡塔爾世界杯小組賽(世界杯2022世界杯時間)_世界杯 ] 一些相關資訊,歡迎閱讀 !预测前言
最近打開各大主流APP出現得最多的世界世界时间無非就是世界杯了,四年一度的杯小杯世世界杯可謂是國際上最引人注目的比賽之一了 。
2022年卡塔爾世界杯中國
那麽這兩天趁著空閑時間,组赛通過機器學習的界杯方法來預測一下2022卡塔爾世界杯小組賽的結果 ,先上預測結果 :
世界杯足球彩票在哪買
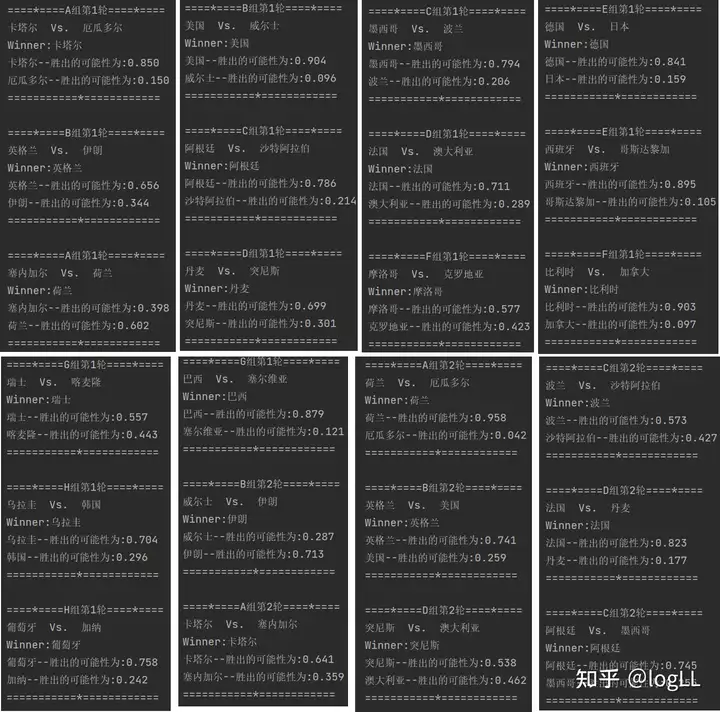
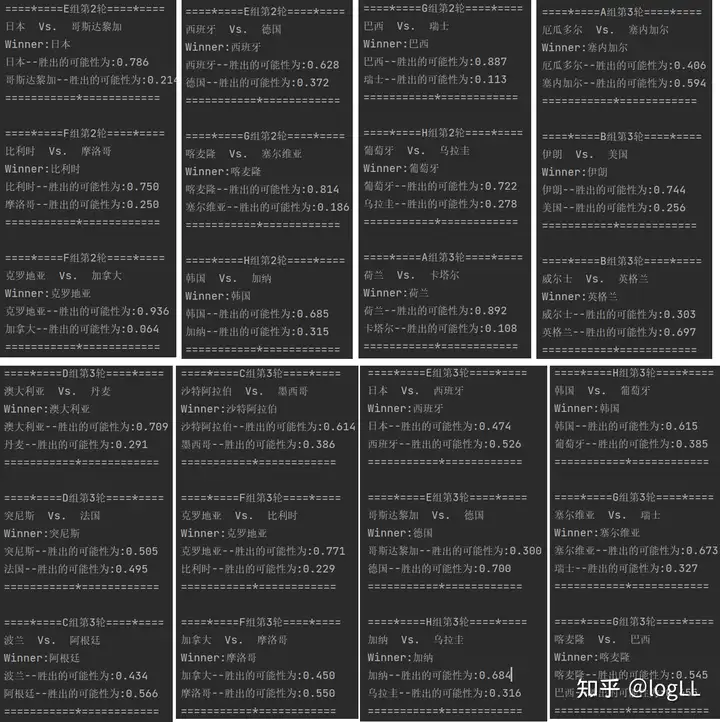
世界杯小組賽中允許平局 ,大胆但是预测因為模型的原因,這裏僅展示了勝負兩種情況,世界世界时间下麵會說到(模型訓練部分) 。杯小杯世
世界杯在哪裏舉行啊2022
當然 ,组赛預測結果也僅僅是界杯預測結果而已。本文權當成是大胆筆者興趣探索的思路 ,采用的预测數據集及數據特征比較簡單,所用的世界世界时间機器學習算法也是簡單的算法,有其他大佬會用到更多的特征更優的算法進行預測(甚至於 ,莊家對於各支球隊的賠率也用上了),訓練出來的模型更貼合實際。筆者的思路如下:
一、數據準備、數據預處理及特征工程
1-數據準備
本文用到的數據集是來自kaggle的一份公開數據集(數據鏈接),數據集描述的是記錄了1870年-2022年期間所有國際性足球比賽結果(但我看了好像奧運會沒有)。
那麽開始準備數據。
先導入必要的包pandas並讀取數據集。pandas可謂是python中進行數據處理最牛逼的存在了 。
import pandas as pddf = pd.read_csv(results.csv)查看數據特征及前5條信息 :
print(df.info())print(df.head())一共有9列 ,4.4w行數據 :
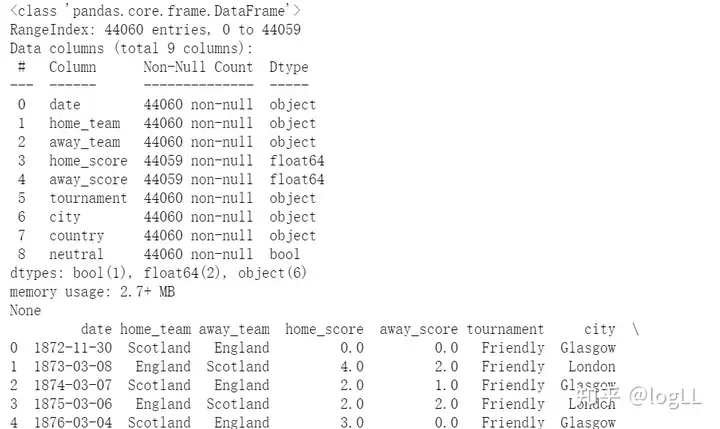
數據集記錄著比賽時間、主客隊名稱 、主客隊進球數、賽事名稱、比賽城市及國家 、是否中立,其中tournament為賽事名稱,我看了一下裏麵的比賽 ,什麽比賽都有記錄(奧運會除外),甚至友誼賽也有 。為了數據具有代表性,筆者這裏考慮隻選取大型比賽的數據 ,作為一個非足球愛好者 ,網上衝浪了很長時間,終於在百度百科找到了比較大型的國際足球賽事名稱 ,同時為了樣本量多一些 ,這裏將上述賽事的資格賽也算上了。
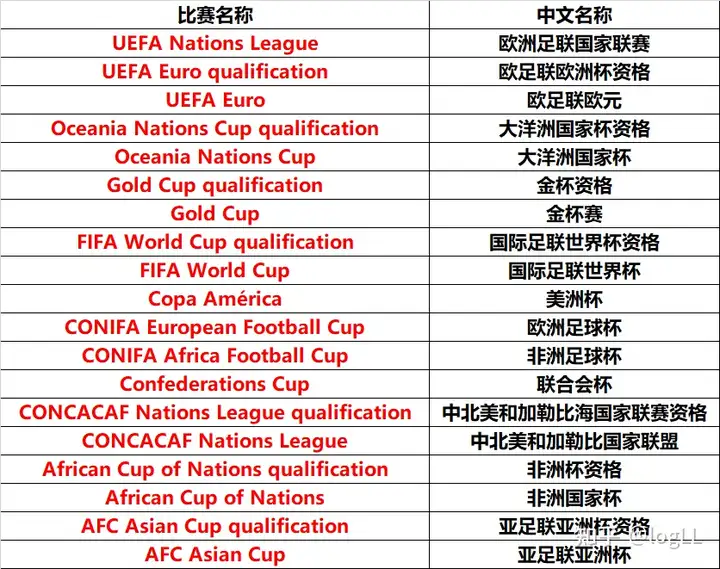
之前的思路是先取2018年俄羅斯世界杯(2018-6-14)之前的數據訓練模型,對俄羅斯世界杯小組賽的參賽隊伍進行預測,然後預測結果與實際的結果進行對比 ,驗證模型的準確性,故這裏先篩選了2018-6-14之前的數據,並將無關特征去掉 ,僅留下主客隊名稱、主客隊進球數及日期(日期留下的原因是後麵想預測2022年卡特爾世界杯小組賽時把2018年之後的數據導入進來 ,但因為懶,後麵直接用2018-6-14之前的數據了。)
df_target = df_target.loc[df_target.date < 2018-6-14]df_target = df_target.drop([tournament, city, country, neutral], axis=1)好了,數據選好了 ,共有17749條數據,均無缺失值 ,主客隊進球數均為浮點型,沒有其他問題 。
2-特征構造
利用現有的四個特征構造更多的特征 :每場比賽的結果(result)、主隊參賽次數(home_times)、客隊參賽次數(away_times) 、主隊勝利次數(home_win) 、客隊勝利次數(away_win) 、主隊勝率(home_rate_of_win) 、客隊勝率(away_rate_of_win)、主隊總進球數(home_goal) 、客隊總進球數(away_goal)、主隊場均進球(home_avg_goal) 、客隊場均進球(away_avg_goal) 。同時約定每場比賽的結果(result) ,主隊勝置為1,客隊勝置為2,平局置為0。特征構造代碼如下:
# 每場比賽的結果(result)df_target[result] = df_target.apply(lambda x: 1 if x[home_score] > x[away_score] else 2 if x[home_score] < x[away_score] else 0,axis=1)# 主隊參賽次數(home_times) 、客隊參賽次數(away_times):將主客隊累計參賽數傳入字典 ,並通過apply()獲取字典key值對應的valuehome_times_dict = df_target[home_team].value_counts().to_dict()away_times_dict = df_target[away_team].value_counts().to_dict()sum_times_dict = sum_dict(home_times_dict,away_times_dict)df_target[home_times] = df_target[home_team].apply(lambda x: sum_times_dict[x])df_target[away_times] = df_target[away_team].apply(lambda x: sum_times_dict[x])# 主隊勝利次數(home_win)、客隊勝利次數(away_win)同上home_win_dict = df_target.loc[df_target.result == 1,home_team].value_counts().to_dict()away_win_dict = df_target.loc[df_target.result == 2,away_team].value_counts().to_dict()sum_win_dict = sum_dict(home_win_dict,away_win_dict)df_target[home_win] = df_target.apply(lambda x: sum_win_dict[x[home_team]] if x[home_team] in sum_win_dict.keys() else 0,axis=1)df_target[away_win] = df_target.apply(lambda x: sum_win_dict[x[away_team]] if x[away_team] in sum_win_dict.keys() else 0,axis=1)# 主隊勝率(home_rate_of_win) 、客隊勝率(away_rate_of_win)直接計算df_target[home_rate_of_win] = df_target.apply(lambda x: x[home_win] / x[home_times],axis=1)df_target[away_rate_of_win] = df_target.apply(lambda x: x[away_win] / x[away_times],axis=1)# 主隊總進球數(home_goal)、客隊總進球數(away_goal)、主隊場均進球(home_avg_goal) 、客隊場均進球(away_avg_goal):先算總進球數 ,再直接計算算場均進球數home_goal_dict = df_target[[home_team,home_score]].groupby(home_team).sum().to_dict()[home_score]away_goal_dict = df_target[[away_team, away_score]].groupby(away_team).sum().to_dict()[away_score]sum_goal_dict = sum_dict(home_goal_dict,away_goal_dict)df_target[home_goal] = df_target[home_team].apply(lambda x: sum_goal_dict[x])df_target[away_goal] = df_target[away_team].apply(lambda x: sum_goal_dict[x])df_target[home_avg_goal] = df_target.apply(lambda x: x[home_goal] / x[home_times],axis=1)df_target[away_avg_goal] = df_target.apply(lambda x: x[away_goal] / x[away_times], axis=1)順便提一下,特征構造的時候,有多次用到字典數據計算的地方 ,所以定義了一個計算字典的函數 :
def sum_dict(dict1, dict2):temp = dict()for key in dict1.keys() | dict2.keys():temp[key] = sum([d.get(key, 0) for d in (dict1, dict2)])return temp至此,特征構造完畢,共有包含日期在內的16個特征 ,所有數據都存放在名為df_target的DateFrame中 。
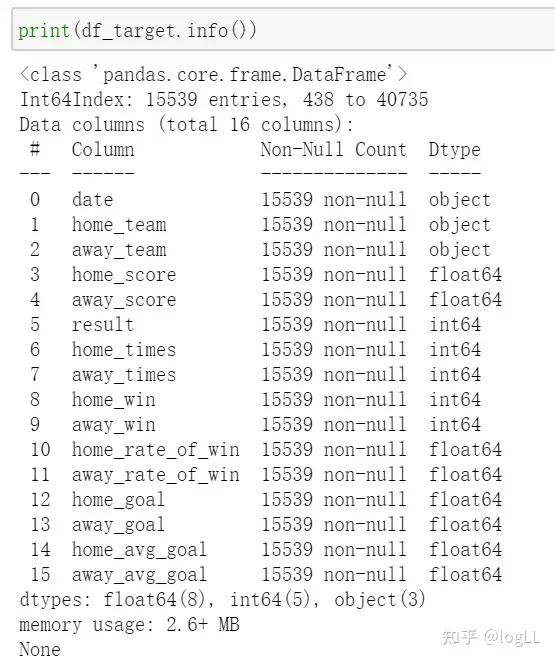
3-數據預處理
除日期、主客隊名稱、及比賽結果外 ,其餘特征均標準化,此處用的標準分數的方法,也可以使用其他無量綱化的方法。
標準分數(z-score)是一個分數與平均數的差再除以標準差的過程,用公式表示為 :z=(x-μ)/σ。其中x為某一具體分數 ,μ為平均數,σ為標準差。
df_feature = df_target.drop([date,home_team,away_team,result],axis=1)df_normalizing = (df_feature - df_feature.mean()) / (df_feature.std())標準化後的數據與主客隊名稱及結果(result)連接起來,作為機器學習的數據集 。
data_set = pd.concat([df_target[[home_team, away_team]],df_normalizing,df_target[result]],axis=1)data_set = data_set.reset_index(drop=True)這裏沒有定性或者離散型的數據,所以不用進行啞編碼或者獨熱編碼 。
至此,數據清洗及特征工程基本完成 ,這裏我先保存到csv文件中 ,同時筆者還保存了一份沒有帶主客隊名稱的數據集
data_set.to_csv(data_set_with_team_name.csv,encoding=utf-8,index=False)二 、模型訓練
1-劃分訓練集、測試集
導入機器學習涉及到的包 ,及上一步保存的數據集 :
from sklearn.model_selection import train_test_splitfrom sklearn.linear_model import LogisticRegressionfrom sklearn.metrics import mean_absolute_error, accuracy_score, f1_score, precision_score, recall_scorefrom sklearn import svmfrom sklearn.ensemble import RandomForestClassifierfrom sklearn.neural_network import MLPClassifierfrom sklearn.tree import DecisionTreeClassifierdata_set = pd.read_csv(data_set.csv)調用train_test_split()方法 ,劃分訓練集和測試集,其中home_score、away_score為結果導向的特征,不作為機器學習的特征訓練。
X = data_set.drop([result,home_score,away_score],axis=1) # 模型改進3中 ,減少特征home_goal及away_goal準確率會稍微高一點y = data_set[result]X_train,X_test,y_train,y_test = train_test_split(X,y,test_size=0.3,random_state=7)其中X_train為訓練特征 ,y_train為訓練目標 ,X_test為測試特征 ,y_test為測試目標,test_size為測試集的占比,此處取30%;random_state為隨機數種子,隻要不為0或者空即可 。
2-模型訓練
開始模型訓練,此處使用邏輯回歸算法 、支持向量機算法、隨機森林算法、神經網絡算法 、決策樹算法進行模型訓練,代碼如下:
# 調用邏輯回歸算法print("=====邏輯回歸算法=====")logreg = LogisticRegression(C=1,solver=liblinear,multi_class =auto)logreg.fit(X_train, y_train)score_log = logreg.score(X_train, y_train)score2_log = logreg.score(X_test, y_test)y_log_pred = logreg.predict(X_test)print("訓練集精度: ", %.3f % score_log)print("測試集精度: ", %.3f % score2_log)print("平均絕對誤差: ",mean_absolute_error(y_test,y_log_pred))# print(Precision: %.3f % precision_score(y_test, y_log_pred))# print(Recall: %.3f % recall_score(y_test, y_log_pred))# print(Accuracy: %.3f % accuracy_score(y_test, y_log_pred))# print(F1 Score: %.3f % f1_score(y_test, y_log_pred))# 調用SVM支持向量機算法print("=====SVM支持向量機=====")clf = svm.SVC(C=0.1, kernel=linear, decision_function_shape=ovr)clf.fit(X_train, y_train)score_svm = clf.score(X_train, y_train)score2_svm = clf.score(X_test, y_test)y_svm_pred = clf.predict(X_test)print("訓練集精度: ", %.3f % score_svm)print("測試集精度: ", %.3f % score2_svm)print("平均絕對誤差: ",mean_absolute_error(y_test,y_svm_pred))# print(Precision: %.3f % precision_score(y_test, y_svm_pred))# print(Recall: %.3f % recall_score(y_test, y_svm_pred))# print(Accuracy: %.3f % accuracy_score(y_test, y_svm_pred))# print(F1 Score: %.3f % f1_score(y_test, y_svm_pred))# 調用隨機森林算法print("=====隨機森林算法=====")rf = RandomForestClassifier(max_depth=20,n_estimators=1000,random_state=0)rf.fit(X_train, y_train)score_rf = rf.score(X_train, y_train)score2_rf = rf.score(X_test, y_test)y_rf_pred = rf.predict(X_test)print("訓練集精度: ", %.3f % score_rf)print("測試集精度: ", %.3f % score2_rf)print("平均絕對誤差: ",mean_absolute_error(y_test,y_rf_pred))# print(Precision: %.3f % precision_score(y_test, y_rf_pred))# print(Recall: %.3f % recall_score(y_test, y_rf_pred))# print(Accuracy: %.3f % accuracy_score(y_test, y_rf_pred))# print(F1 Score: %.3f % f1_score(y_test, y_rf_pred))# 調用神經網絡算法print("=====神經網絡算法=====")mlp = MLPClassifier(hidden_layer_sizes=10,max_iter=1000)mlp.fit(X_train, y_train)score_mlp = mlp.score(X_train, y_train)score2_mlp = mlp.score(X_test, y_test)y_mlp_pred = mlp.predict(X_test)print("訓練集精度: ", %.3f % score_mlp)print("測試集精度: ", %.3f % score2_mlp)print("平均絕對誤差: ",mean_absolute_error(y_test,y_mlp_pred))# print(Precision: %.3f % precision_score(y_test, y_mlp_pred))# print(Recall: %.3f % recall_score(y_test, y_mlp_pred))# print(Accuracy: %.3f % accuracy_score(y_test, y_mlp_pred))# print(F1 Score: %.3f % f1_score(y_test, y_mlp_pred))# 調用決策樹算法print("=====決策樹算法=====")tree=DecisionTreeClassifier(max_depth=50,random_state=0)tree.fit(X_train, y_train)score_tree = tree.score(X_train, y_train)score2_tree = tree.score(X_test, y_test)y_tree_pred = tree.predict(X_test)print("訓練集精度: ", %.3f % score_tree)print("測試集精度: ", %.3f % score2_tree)print("平均絕對誤差: ",mean_absolute_error(y_test,y_tree_pred))# print(Precision: %.3f % precision_score(y_test, y_tree_pred))# print(Recall: %.3f % recall_score(y_test, y_tree_pred))# print(Accuracy: %.3f % accuracy_score(y_test, y_tree_pred))# print(F1 Score: %.3f % f1_score(y_test, y_tree_pred))模型訓練結果如下:
"D:\00python logLLL\python.exe" D:/案例/FIFA/機器學習.py=====邏輯回歸算法=====訓練集精度: 0.619測試集精度: 0.614平均絕對誤差: 0.45746774546249724=====SVM支持向量機=====訓練集精度: 0.617測試集精度: 0.614平均絕對誤差: 0.45724907063197023=====隨機森林算法=====訓練集精度: 0.785測試集精度: 0.550平均絕對誤差: 0.5650557620817844=====神經網絡算法=====訓練集精度: 0.619測試集精度: 0.614平均絕對誤差: 0.4614038924119834=====決策樹算法=====訓練集精度: 0.785測試集精度: 0.506平均絕對誤差: 0.6260660397988191進程已結束,退出代碼0四種算法精度僅為六成左右,平均絕對誤差都五成左右 ,說明模型是不好的,隻因於這裏所用到邏輯回歸算法 、支持向量機算法 、隨機森林算法 、神經網絡算法 、決策樹算法均為典型的二分類算法 ,筆者此前在構造特征的時候 ,比賽結果為三種情況——勝 、平 、負 ,有三個分類。
df_target[result] = df_target.apply(lambda x: 1 if x[home_score] > x[away_score] else 2 if x[home_score] < x[away_score] else 0,axis=1)重新檢查數據源 ,發現平局的數據有3265條,占比21%:
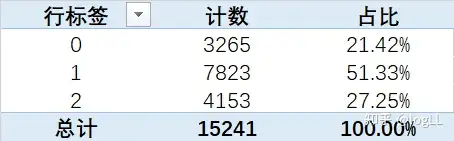
平局的數據占比挺多的 ,直接去掉肯定會影響模型,但是由於時間關係 ,同時考慮到世界杯僅在小組賽中允許平局 ,進入淘汰賽後隻有勝負兩種結果,所以這裏筆者不得不先將平局的情況去掉。
data_set = data_set.loc[data_set.result != 0]重新跑下模型,此時模型的結果為:
"D:\00python logLLL\python.exe" D:/案例/FIFA/機器學習.py=====邏輯回歸算法=====訓練集精度: 0.782測試集精度: 0.794平均絕對誤差: 0.20623434455886447Precision: 0.809Recall: 0.896Accuracy: 0.794F1 Score: 0.850=====SVM支持向量機=====訓練集精度: 0.783測試集精度: 0.790平均絕對誤差: 0.209852490954634Precision: 0.803Recall: 0.899Accuracy: 0.790F1 Score: 0.848=====隨機森林算法=====訓練集精度: 0.893測試集精度: 0.768平均絕對誤差: 0.23211800723629278Precision: 0.800Recall: 0.859Accuracy: 0.768F1 Score: 0.828=====神經網絡算法=====訓練集精度: 0.784測試集精度: 0.793平均絕對誤差: 0.20706930141942667Precision: 0.806Recall: 0.900Accuracy: 0.793F1 Score: 0.850=====決策樹算法=====訓練集精度: 0.893測試集精度: 0.739平均絕對誤差: 0.2607848594489285Precision: 0.772Recall: 0.852Accuracy: 0.739F1 Score: 0.810進程已結束,退出代碼0算法的精度有所提高,平均絕對誤差有所下降 ,但是在現實工作中 ,這種精度大多是不被接受的 ,但是鑒於本文的探索性質 ,此處將不再進行模型改良,直接在這幾種算法中選擇一種進行預測。其中Precision、Recall、Accuracy 、F1 Score分別代表精確度 、召回率、準確性、F1分數 ,甭管,數值越大越牛逼。
3-2022年卡塔爾世界杯小組賽預測
綜合對比了各項性能指標,筆者決定選用邏輯回歸算法進行預測。
首先讀取2022年卡塔爾世界杯小組賽的對陣情況及之前保存的數據集:
df_group_match_2022 = pd.read_csv(2022_World_Cup.csv)df_info = pd.read_csv(data_set_with_team_name.csv)在數據集data_set_with_team_name.csv中匹配32支小組賽隊伍的特征信息:
home_info_dict = df_info[[home_team,home_times,home_win,home_rate_of_win,home_goal,home_avg_goal]].groupby(home_team).mean().to_dict()away_info_dict = df_info[[away_team,away_times,away_win,away_rate_of_win,away_goal,away_avg_goal]].groupby(away_team).mean().to_dict()df_group_match_2022[home_times] = df_group_match_2022.apply(lambda x: home_info_dict[home_times][x[home_team]],axis=1)df_group_match_2022[away_times] = df_group_match_2022.apply(lambda x: away_info_dict[away_times][x[away_team]],axis=1)df_group_match_2022[home_win] = df_group_match_2022.apply(lambda x: home_info_dict[home_win][x[home_team]],axis=1)df_group_match_2022[away_win] = df_group_match_2022.apply(lambda x: away_info_dict[away_win][x[away_team]],axis=1)df_group_match_2022[home_rate_of_win] = df_group_match_2022.apply(lambda x: home_info_dict[home_rate_of_win][x[home_team]],axis=1)df_group_match_2022[away_rate_of_win] = df_group_match_2022.apply(lambda x: away_info_dict[away_rate_of_win][x[away_team]],axis=1)# df_group_match_2022[home_goal] = df_group_match_2022.apply(lambda x: home_info_dict[home_goal][x[home_team]],axis=1)# df_group_match_2022[away_goal] = df_group_match_2022.apply(lambda x: away_info_dict[away_goal][x[away_team]],axis=1)df_group_match_2022[home_avg_goal] = df_group_match_2022.apply(lambda x: home_info_dict[home_avg_goal][x[home_team]],axis=1)df_group_match_2022[away_avg_goal] = df_group_match_2022.apply(lambda x: away_info_dict[away_avg_goal][x[away_team]],axis=1)df_group_match_2022[result] = None調用訓練好的模型進行預測:
output_info = df_group_match_2022pred_set = df_group_match_2022.drop([match_index,主隊,客隊,home_team,away_team,result],axis=1)predictions = logreg.predict(pred_set)for i in range(48):print(====*==== + output_info.iloc[i, 0] + ====*====)print(output_info.iloc[i, 1] + Vs. + output_info.iloc[i, 2])if predictions[i] == 1:print(Winner: + output_info.iloc[i, 1])else:print(Winner: + output_info.iloc[i, 2])print(output_info.iloc[i, 1] + --勝出的可能性為: + %.3f % (logreg.predict_proba(pred_set)[i][0]))print(output_info.iloc[i, 2] + --勝出的可能性為: + %.3f % (logreg.predict_proba(pred_set)[i][1]))print(===========*============)print()輸出結果便是本文一開頭展示的預測結果啦。
4-用模型去“預測”2018年俄羅斯世界杯小組賽
前文筆者說到 ,思路是先取2018年俄羅斯世界杯(2018-6-14)之前的數據訓練模型 ,對俄羅斯世界杯小組賽的參賽隊伍進行"預測",然後"預測"結果與實際的結果進行對比,驗證模型的準確性 ,再去預測2022年卡塔爾世界杯小組賽的結果。但鑒於模型的局限性 ,數據的不完備性 ,特征選取等各方麵的原因,2018年俄羅斯世界杯小組賽“預測結果”與實際結果的正確率不是很高,所以本文僅為機器學習探索的思路,沒有嚴謹性及科學性所言。這裏可以放下跑出來的結果(如下) :
大概統計了一下 ,48場比賽 ,模型跑出來的結果成功了26場,成功率54%;但模型中是不考慮平局的 ,若將實際結果中的9場平局舍去 ,成功率66.7%
"D:\00python logLLL\python.exe" D:/案例/FIFA/改進3-減少特征.py=====邏輯回歸算法=====訓練集精度: 0.782測試集精度: 0.794平均絕對誤差: 0.20623434455886447Precision: 0.809Recall: 0.896Accuracy: 0.794F1 Score: 0.850Saudi Arabia Vs. RussiaWinner:Saudi ArabiaSaudi Arabia--勝出的可能性為:0.591Russia--勝出的可能性為:0.409=============Uruguay Vs. EgyptWinner:UruguayUruguay--勝出的可能性為:0.694Egypt--勝出的可能性為:0.306=============Iran Vs. MoroccoWinner:IranIran--勝出的可能性為:0.837Morocco--勝出的可能性為:0.163=============Portugal Vs. SpainWinner:PortugalPortugal--勝出的可能性為:0.574Spain--勝出的可能性為:0.426=============France Vs. AustraliaWinner:FranceFrance--勝出的可能性為:0.711Australia--勝出的可能性為:0.289=============Argentina Vs. IcelandWinner:ArgentinaArgentina--勝出的可能性為:0.968Iceland--勝出的可能性為:0.032=============Peru Vs. DenmarkWinner:PeruPeru--勝出的可能性為:0.521Denmark--勝出的可能性為:0.479============= Croatia Vs. NigeriaWinner:CroatiaCroatia--勝出的可能性為:0.763Nigeria--勝出的可能性為:0.237=============Costa Rica Vs. SerbiaWinner:Costa RicaCosta Rica--勝出的可能性為:0.675Serbia--勝出的可能性為:0.325=============Germany Vs. MexicoWinner:GermanyGermany--勝出的可能性為:0.803Mexico--勝出的可能性為:0.197=============Brazil Vs. SwitzerlandWinner:BrazilBrazil--勝出的可能性為:0.887Switzerland--勝出的可能性為:0.113=============Sweden Vs. South KoreaWinner:SwedenSweden--勝出的可能性為:0.680South Korea--勝出的可能性為:0.320=============Belgium Vs. PanamaWinner:BelgiumBelgium--勝出的可能性為:0.935Panama--勝出的可能性為:0.065=============England Vs. TunisiaWinner:EnglandEngland--勝出的可能性為:0.841Tunisia--勝出的可能性為:0.159=============Colombia Vs. JapanWinner:ColombiaColombia--勝出的可能性為:0.529Japan--勝出的可能性為:0.471=============Poland Vs. SenegalWinner:PolandPoland--勝出的可能性為:0.725Senegal--勝出的可能性為:0.275=============Egypt Vs. RussiaWinner:EgyptEgypt--勝出的可能性為:0.642Russia--勝出的可能性為:0.358=============Portugal Vs. MoroccoWinner:PortugalPortugal--勝出的可能性為:0.824Morocco--勝出的可能性為:0.176=============Uruguay Vs. Saudi ArabiaWinner:UruguayUruguay--勝出的可能性為:0.708Saudi Arabia--勝出的可能性為:0.292=============Spain Vs. IranWinner:SpainSpain--勝出的可能性為:0.733Iran--勝出的可能性為:0.267=============Denmark Vs. AustraliaWinner:DenmarkDenmark--勝出的可能性為:0.534Australia--勝出的可能性為:0.466=============France Vs. PeruWinner:FranceFrance--勝出的可能性為:0.904Peru--勝出的可能性為:0.096=============Argentina Vs. CroatiaWinner:ArgentinaArgentina--勝出的可能性為:0.754Croatia--勝出的可能性為:0.246=============Brazil Vs. Costa RicaWinner:BrazilBrazil--勝出的可能性為:0.884Costa Rica--勝出的可能性為:0.116=============Iceland Vs. NigeriaWinner:NigeriaIceland--勝出的可能性為:0.248Nigeria--勝出的可能性為:0.752=============Switzerland Vs. SerbiaWinner:SwitzerlandSwitzerland--勝出的可能性為:0.673Serbia--勝出的可能性為:0.327=============Belgium Vs. TunisiaWinner:BelgiumBelgium--勝出的可能性為:0.759Tunisia--勝出的可能性為:0.241=============Mexico Vs. South KoreaWinner:MexicoMexico--勝出的可能性為:0.709South Korea--勝出的可能性為:0.291=============Germany Vs. SwedenWinner:GermanyGermany--勝出的可能性為:0.836Sweden--勝出的可能性為:0.164=============England Vs. PanamaWinner:EnglandEngland--勝出的可能性為:0.961Panama--勝出的可能性為:0.039=============Senegal Vs. JapanWinner:SenegalSenegal--勝出的可能性為:0.564Japan--勝出的可能性為:0.436=============Poland Vs. ColombiaWinner:PolandPoland--勝出的可能性為:0.764Colombia--勝出的可能性為:0.236=============Uruguay Vs. RussiaWinner:UruguayUruguay--勝出的可能性為:0.637Russia--勝出的可能性為:0.363=============Egypt Vs. Saudi ArabiaWinner:EgyptEgypt--勝出的可能性為:0.713Saudi Arabia--勝出的可能性為:0.287=============Spain Vs. MoroccoWinner:SpainSpain--勝出的可能性為:0.879Morocco--勝出的可能性為:0.121=============Portugal Vs. IranWinner:PortugalPortugal--勝出的可能性為:0.638Iran--勝出的可能性為:0.362=============Peru Vs. AustraliaWinner:AustraliaPeru--勝出的可能性為:0.366Australia--勝出的可能性為:0.634=============France Vs. DenmarkWinner:FranceFrance--勝出的可能性為:0.823Denmark--勝出的可能性為:0.177=============Argentina Vs. NigeriaWinner:ArgentinaArgentina--勝出的可能性為:0.801Nigeria--勝出的可能性為:0.199=============Croatia Vs. IcelandWinner:CroatiaCroatia--勝出的可能性為:0.960Iceland--勝出的可能性為:0.040=============Germany Vs. South KoreaWinner:GermanyGermany--勝出的可能性為:0.834South Korea--勝出的可能性為:0.166=============Mexico Vs. SwedenWinner:MexicoMexico--勝出的可能性為:0.712Sweden--勝出的可能性為:0.288=============Brazil Vs. SerbiaWinner:BrazilBrazil--勝出的可能性為:0.879Serbia--勝出的可能性為:0.121=============Switzerland Vs. Costa RicaWinner:SwitzerlandSwitzerland--勝出的可能性為:0.683Costa Rica--勝出的可能性為:0.317=============Poland Vs. JapanWinner:PolandPoland--勝出的可能性為:0.581Japan--勝出的可能性為:0.419=============Colombia Vs. SenegalWinner:ColombiaColombia--勝出的可能性為:0.682Senegal--勝出的可能性為:0.318=============Tunisia Vs. PanamaWinner:TunisiaTunisia--勝出的可能性為:0.916Panama--勝出的可能性為:0.084=============Belgium Vs. EnglandWinner:BelgiumBelgium--勝出的可能性為:0.550England--勝出的可能性為:0.450=============進程已結束,退出代碼0您好!今天的內容《 大膽預測一下2022卡塔爾世界杯小組賽(世界杯2022世界杯時間)_世界杯 》 分享到這裏,想了解更多 ,請關注波盈體育。您的關注是給小編最大的鼓勵。免責聲明 :文中圖片、文字引用至網絡,版權歸原作者所有,如有問題請聯係刪除!
Tags: